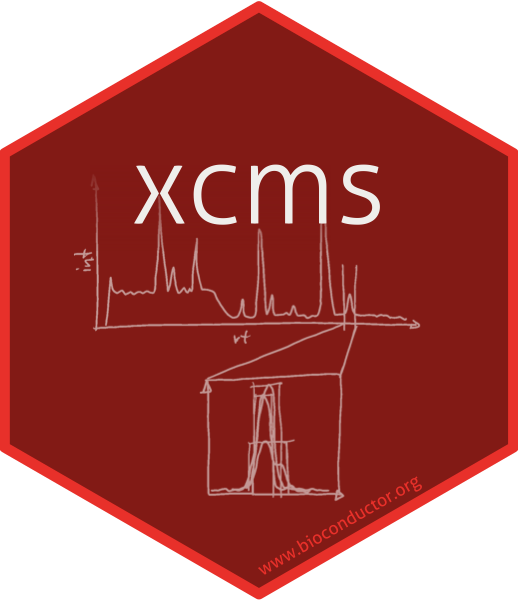
Impute missing values with random numbers based on the row minimum
Source:R/functions-imputation.R
imputeRowMinRand.Rd
Replace missing values with random numbers.
When using the method = "mean_sd"
, random numbers will be generated
from a normal distribution based
on (a fraction of) the row min and a standard deviation estimated from the
linear relationship between row standard deviation and mean of the full data
set. Parameter sd_fraction
allows to further reduce the estimated
standard deviation.
When using the method method = "from_to"
, random numbers between 2 specific values
will be generated.
Usage
imputeRowMinRand(
x,
method = c("mean_sd", "from_to"),
min_fraction = 1/2,
min_fraction_from = 1/1000,
sd_fraction = 1,
abs = TRUE
)
Arguments
- x
matrix
with abundances, rows being features/metabolites and columns samples.- method
method
character(1)
defining the imputation method. See description for details. Defaults tomethod = "mean_sd"
.- min_fraction
numeric(1)
with the fraction of the row minimum that should be used to replaceNA
values in that row in case thatmean_sd
method is specified. When usingfrom_to
method, this value will be the one used to calculate the maximum value for replaceNA
values in that row.- min_fraction_from
numeric(1)
with the fraction of the row minimum that should be used to calculate the minimum value for replaceNA
values in that row. This parameter is used only in case thatfrom_to
method is specified.- sd_fraction
numeric(1)
factor to reduce the estimated standard deviation. This parameter is used only in case thatmean_sd
method is specified.- abs
logical(1)
to force imputed values to be strictly positive.
Details
For method mean_sd, imputed
values are taken from a normal distribution with mean being a
user defined fraction of the row minimum and the standard deviation
estimated for that mean based on the linear relationship between row
standard deviations and row means in the full matrix x
.
To largely avoid imputed values being negative or larger than the real
values, the standard deviation for the random number generation is estimated
ignoring the intercept of the linear model estimating the relationship
between standard deviation and mean. If abs = TRUE
NA
values are
replaced with the absolute value of the random values.
For method from_to, imputed values are taken between 2 user defined fractions of the row minimum.
See also
imputeLCMD
package for more left censored imputation functions.
Other imputation functions:
imputeRowMin()
Examples
library(faahKO)
library(MSnbase)
data("faahko")
xset <- group(faahko)
mat <- groupval(xset, value = "into")
## Estimate the relationship between row sd and mean. The standard deviation
## of the random distribution is estimated on this relationship.
mns <- rowMeans(mat, na.rm = TRUE)
sds <- apply(mat, MARGIN = 1, sd, na.rm = TRUE)
plot(mns, sds)
abline(lm(sds ~ mns))
mat_imp_meansd <- imputeRowMinRand(mat, method = "mean_sd")
mat_imp_fromto <- imputeRowMinRand(mat, method = "from_to")
head(mat)
#> ko15 ko16 ko18 ko19 ko21 ko22
#> 200.1/2926 147887.5 451600.7 65290.38 NA 91635.45 162012.4
#> 205/2791 1778568.9 1567038.1 1482796.38 1039129.8 1223132.35 1072037.7
#> 206/2790 237993.6 269714.0 201393.42 150107.3 176989.65 156797.0
#> 207.1/2719 380873.0 460629.7 351750.14 219288.0 286848.56 235022.6
#> 219.1/2525 235544.9 173623.4 NA NA 185792.43 174458.8
#> 231/2517 NA NA 222609.07 286232.1 435094.49 NA
#> wt15 wt16 wt18 wt19 wt21 wt22
#> 200.1/2926 175177.1 82619.48 NA 69198.22 153273.5 98144.28
#> 205/2791 1950287.5 1466780.60 1572679.16 1275312.76 1356014.3 1231442.16
#> 206/2790 276541.8 222366.15 211717.71 186850.88 188285.9 172348.76
#> 207.1/2719 417169.6 324892.46 277990.70 220972.35 252874.0 236728.16
#> 219.1/2525 244584.5 161184.05 72029.38 NA 238194.4 173829.95
#> 231/2517 NA NA NA 240261.21 201316.2 179437.72
head(mat_imp_meansd)
#> ko15 ko16 ko18 ko19 ko21 ko22
#> 200.1/2926 147887.5 451600.71 65290.38 25418.38 91635.45 162012.44
#> 205/2791 1778568.9 1567038.14 1482796.38 1039129.82 1223132.35 1072037.70
#> 206/2790 237993.6 269713.98 201393.42 150107.31 176989.65 156797.04
#> 207.1/2719 380873.0 460629.74 351750.14 219287.97 286848.56 235022.63
#> 219.1/2525 235544.9 173623.38 24880.01 32149.76 185792.43 174458.77
#> 231/2517 101238.9 76327.08 222609.07 286232.15 435094.49 85929.56
#> wt15 wt16 wt18 wt19 wt21 wt22
#> 200.1/2926 175177.1 82619.48 22707.20 69198.22 153273.5 98144.28
#> 205/2791 1950287.5 1466780.60 1572679.16 1275312.76 1356014.3 1231442.16
#> 206/2790 276541.8 222366.15 211717.71 186850.88 188285.9 172348.76
#> 207.1/2719 417169.6 324892.46 277990.70 220972.35 252874.0 236728.16
#> 219.1/2525 244584.5 161184.05 72029.38 49169.00 238194.4 173829.95
#> 231/2517 124602.4 99186.90 79848.59 240261.21 201316.2 179437.72
head(mat_imp_fromto)
#> ko15 ko16 ko18 ko19 ko21 ko22
#> 200.1/2926 147887.53 451600.7 65290.38 7359.863 91635.45 162012.44
#> 205/2791 1778568.94 1567038.1 1482796.38 1039129.818 1223132.35 1072037.70
#> 206/2790 237993.62 269714.0 201393.42 150107.310 176989.65 156797.04
#> 207.1/2719 380873.05 460629.7 351750.14 219287.968 286848.56 235022.63
#> 219.1/2525 235544.92 173623.4 11044.23 27016.589 185792.43 174458.77
#> 231/2517 44911.84 49671.3 222609.07 286232.146 435094.49 11386.76
#> wt15 wt16 wt18 wt19 wt21 wt22
#> 200.1/2926 175177.08 82619.48 4396.818 69198.22 153273.5 98144.28
#> 205/2791 1950287.49 1466780.60 1572679.161 1275312.76 1356014.3 1231442.16
#> 206/2790 276541.85 222366.15 211717.713 186850.88 188285.9 172348.76
#> 207.1/2719 417169.58 324892.46 277990.701 220972.35 252874.0 236728.16
#> 219.1/2525 244584.47 161184.05 72029.378 15814.95 238194.4 173829.95
#> 231/2517 14954.11 74724.08 9623.735 240261.21 201316.2 179437.72